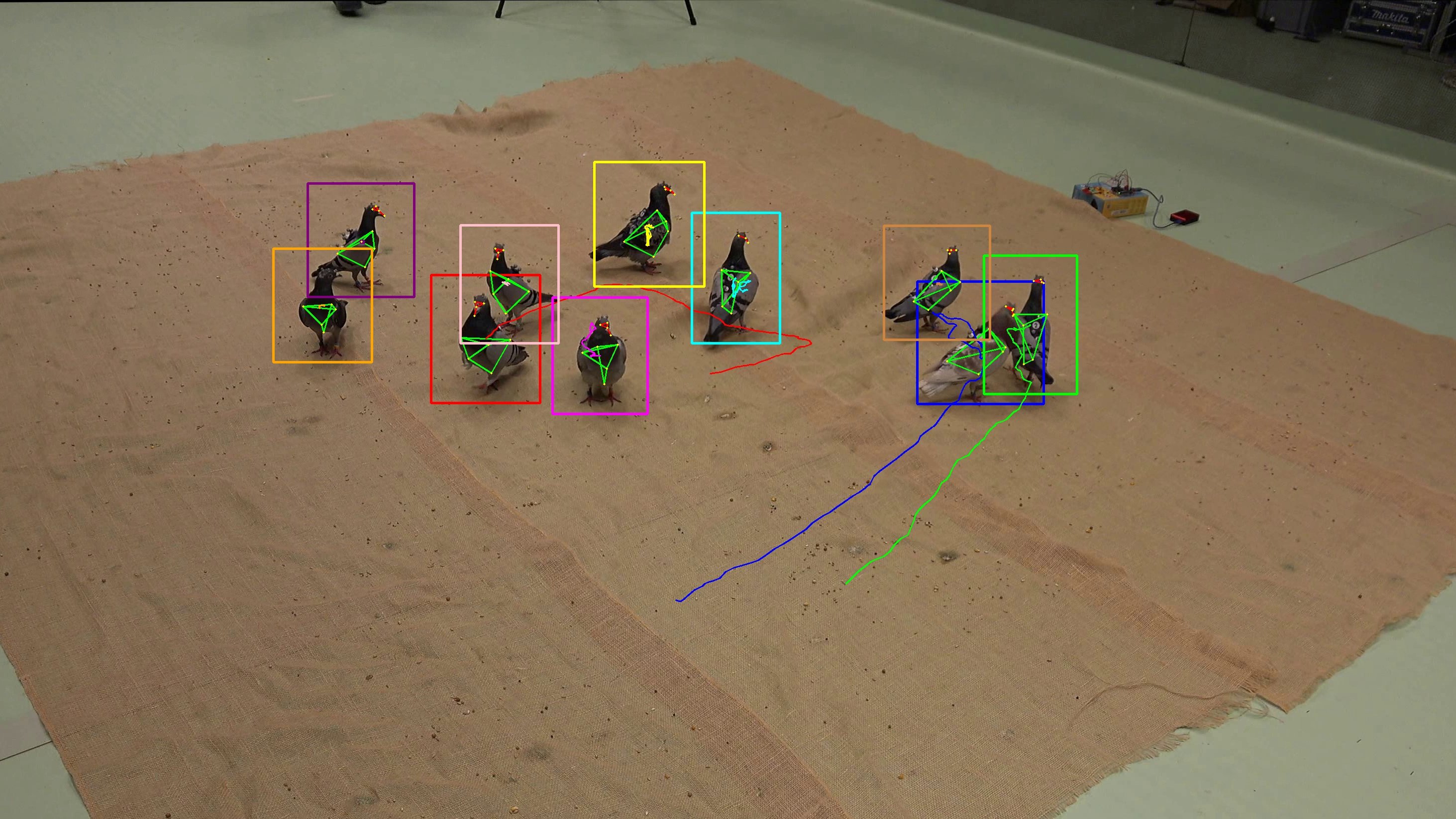
Studying animal behaviour without markers
With a new marker less method it is now possible to track the gaze and fine-scaled behaviours of every individual bird and how that animal moves in the space with others. A research team from the Cluster of Excellence Centre for the Advanced Study of Collective Behaviour (CASCB) at the University of Konstanz developed a dataset to advance behavioural research.
Researchers are still puzzling over how animal collectives behave, but recent advances in machine learning and computer vision are revolutionizing the possibilities of studying animal behaviour. Complex behaviours, like social learning or collective vigilance can be deciphered with new techniques. An interdisciplinary research team from the Cluster of Excellence Centre for the Advanced Study of Collective Behaviour (CASCB) at the University of Konstanz and the Max Planck Institute of Animal Behavior has now succeeded in developing a novel marker less method to track bird postures in 3D just by using camera images. With this method it is possible to record a group of pigeons and identify the gaze and fine-scaled behaviours of every individual bird and how that animal moves in the space with others.
Pushing the research of understanding animal behaviour in 3D ahead
3D-POP stands for 3D posture of pigeons which is a large-scale dataset containing accurate information about 3D position, posture of beak or eyes, and identity for freely moving pigeons. “An animal dataset of such scale and complexity has never been done before,” says Alex Chan, PhD student at the CASCB. “3D-POP is a dataset that will help the community to develop automatic methods to extract position and posture (in 3D) by using machine learning and artificial intelligence. With the dataset, researchers can study collective behaviour of birds by just using at least two video cameras, even in the wild.”
How the data set was developed
The 3D-POP dataset was developed using a motion capture system and around ten pigeons. Only for the development of the dataset was it again necessary that the pigeons wear tiny reflective markers. The markers have a unique geometric configuration to track the individual identities of each bird. “Motion capture cameras compute 3D positions of the reflective markers with sub-millimetre precision,” explains Alex Chan. The 3D data from the motion-capture system is mapped to video images to mark the position of the bird and features like their eyes, nose, or tail.
“The behavioural scoring, also called annotation, is so far mostly done manually. We tried to solve this problem by offering a new solution that produces annotations automatically”, Hemal Naik outlines. “For large scale monitoring, AI systems will use movements and posture information to automatically classify behavioural traits such as aggression, submissive behaviour, or mating display.” But the community needs datasets to build the technology to detect movement, posture, and identify animals in the images. “This is where our method helps because we can produce millions of annotations with a few clicks,” says Naik. Furthermore, the method is a huge step forwards because while the dataset exists markers are no longer needed for studying pigeons with the 3D-POP dataset.
3D-POP bridges the gap between biologists and computer scientists
“3D-POP bridges the gap between biologists and computer scientists, because computer scientists would not have access to animals to create such datasets, and biologists rarely know what type of data computer scientists need to push the development of new methods,” says Alex Chan who has a background in biology with a keen interest in computer science. Within the Cluster of Excellence CASCB, interdisciplinary collaboration is highly asked for. That is why researchers from both fields came together and exchanged their specific needs.
The dataset was released at the Conference on Computer Vision and Pattern Recognition (CVPR) in June 2023 and available via open access so that it can be reused by other researchers. Naik and Chan see two potential application areas: Scientists working with pigeons can directly use the dataset. With at least two cameras they can study the behaviour of multiple freely moving pigeons. The annotation method can be used with other birds or even other animals so that researchers can soon decipher the behaviour of other animals.
Key facts:
- Publication: Hemal Naik, Alex Hoi Hang Chan, Junran Yang, Mathilde Delacoux, Iain D. Couzin, Fumihiro Kano, Máté Nagy; Proceedings of the IEEE/CVF Conference on Computer Vision and Pattern Recognition (CVPR), 2023, pp. 21274-21284.
- The paper was published at the Conference on Computer Vision and Pattern Recognition (CVPR), one of the most prestigious computer science conferences in the world.
- The dataset and code to apply the annotation method to other birds can be found here: github.com/alexhang212/Dataset-3DPOP
- An interdisciplinary study team from the Cluster of Excellence Centre for the Advanced Study of Collective Behaviour (CASCB) and the Max Planck Institute of Animal Behavior with computer scientists, biologists, and comparative psychologists developed a new method for generating large-scale datasets with multiple animals. Alex Chan and Hemal Naik are both first authors of the publication.
- The study was funded by the Cluster of Excellence Centre for the Advanced Study of Collective Behaviour at the University of Konstanz.